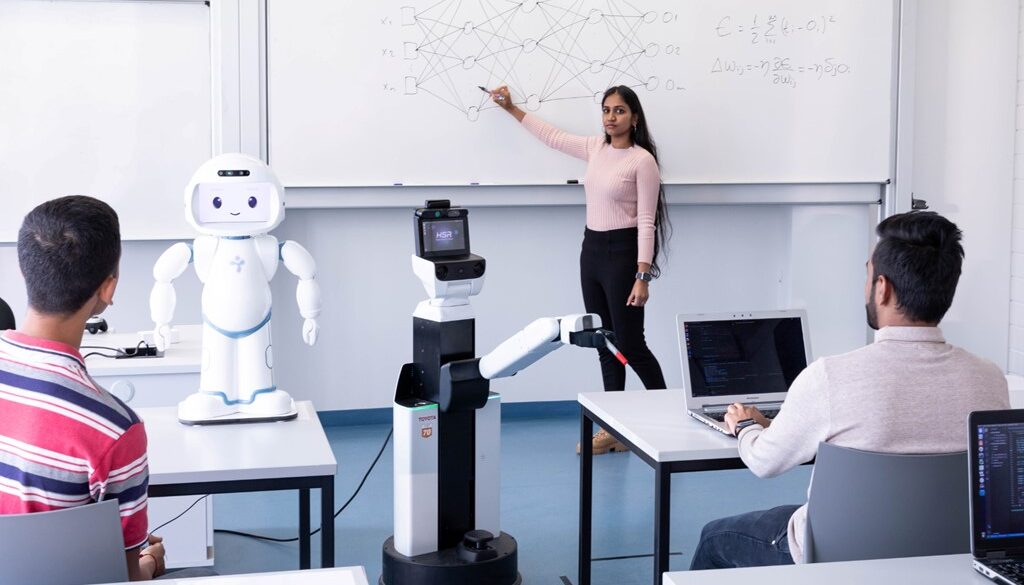
Abstract:
During robot-assisted therapy, therapists often need to partially or fully control the robot, such as through a Wizard-of-Oz protocol. This can make therapeutic sessions tedious, as therapists cannot fully focus on interacting with the person under therapy. In this work, the researchers developed a learning-based behavior model to increase the autonomy of a robot’s decision-making process. They investigated reinforcement learning as a model training technique and compared different reward functions that consider a user’s engagement and activity performance. Various strategies were analyzed to make the learning process more manageable, including i) behavior model training with a learned user model, ii) policy transfer between user groups, and iii) policy learning from expert feedback.
The researchers demonstrated that policy transfer can significantly speed up the policy learning process, although the reward function greatly influences the actions the robot can choose. While the main focus of this paper is the personalization pipeline itself, the learned behavior models were further evaluated in a small-scale real-world feasibility study. Six users participated in a sequence learning game with an assistive robot. The results suggest that learning from guidance may produce the most effective policies in terms of increasing user engagement and game performance. However, a large-scale user study is needed to verify the validity of these findings.
Reference:
Learning-based personalization of robot behavior for robot-assisted therapy, Stolarz et al, Frontiers in Robotics and AI, volume 11, 2024