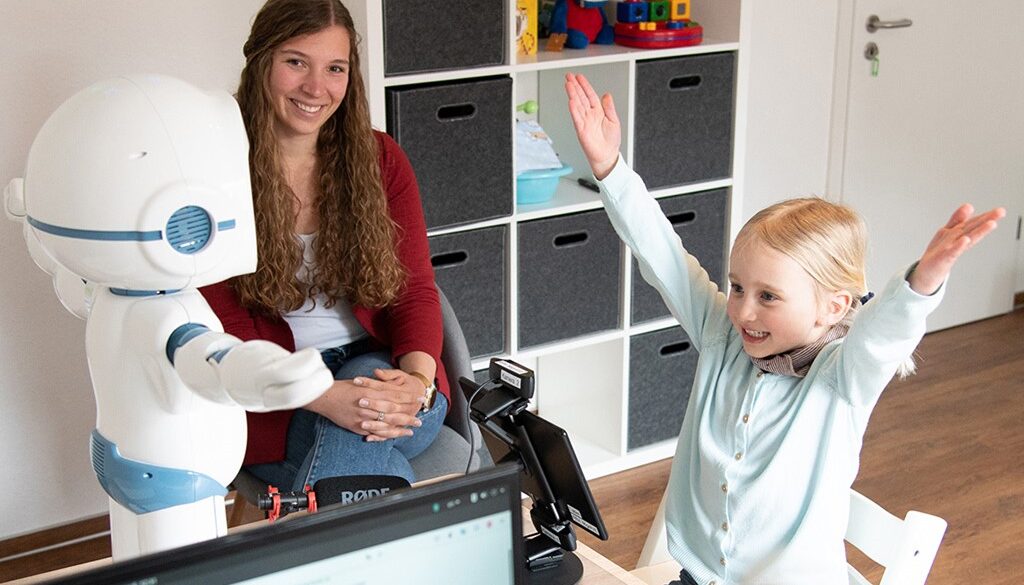
Abstract:
In therapeutic scenarios, robots are often used for imitation activities where the robot demonstrates a motion that the individual under therapy needs to repeat. To enable the incorporation of new types of motions in such activities, the robot must be able to learn motions by observing demonstrations from a human, such as a therapist. This paper investigates an approach for acquiring motions from human skeleton observations collected by a robot-centric RGB-D camera. The learning process involves mapping joint angle positions from the human body to the robot, ensuring self-collisions are prevented by adjusting the angles to safe positions.
The researchers conducted both quantitative and qualitative evaluations of the method. For the quantitative evaluation, the motion reproduction error was assessed through a study in which QTrobot acquired various upper-body dance moves from multiple participants. The qualitative evaluation involved a user study to gauge perceived reproduction accuracy. The quantitative results demonstrate the method’s overall feasibility, despite reproduction quality being affected by noise in the skeleton observations. The qualitative evaluation indicated general satisfaction with the robot’s motions, except for those likely to lead to self-collisions, which were reproduced less accurately.
Reference:
Quiroga, Natalia, Alex Mitrevski, and Paul G. Plögery. “A Study of Demonstration-Based Learning of Upper-Body Motions in the Context of Robot-Assisted Therapy.” 2023 32nd IEEE International Conference on Robot and Human Interactive Communication (RO-MAN). IEEE, 2023.