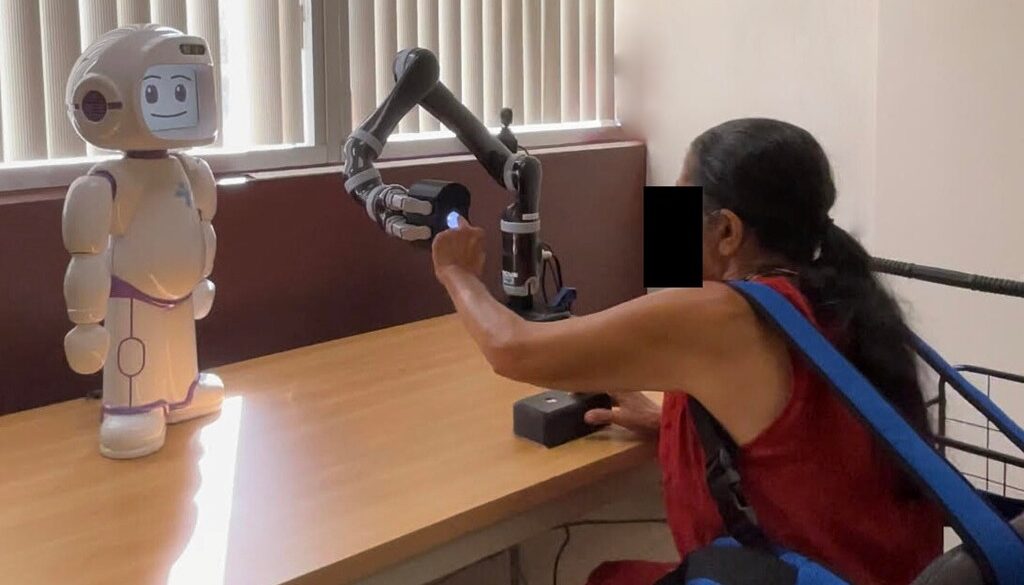
The Challenge: Traditional rehabilitation robots often apply a one-size-fits-all approach to exercise difficulty. However, stroke survivors experience exercise difficulty in very unique ways – what’s hard for one might be easier for another. This can lead to exercises being too challenging (demotivating) or too easy (ineffective).
The Solution: Researchers have developed an innovative method using causal trees to model personalized difficulty of rehabilitation exercises. This approach, evaluated with the LuxAI QTrobot and a robotic arm, learns directly from a user’s performance data to understand exactly why a specific movement is challenging for them.
How it Works with QTrobot:
- Data-Driven Personalization: The QTrobot guides users through reaching exercises while a connected robot arm moves a target. The system records the user’s reach times for various target locations.
- Causal Tree Intelligence: This data is fed into a causal tree model. By comparing the user’s performance to baseline data from neurotypical individuals, the model identifies specific exercise characteristics (like reach direction and height) that significantly impact the user’s difficulty.
- Visualizing Difficulty: The system generates intuitive visualizations, like heatmaps on the user’s workspace, where lighter areas indicate more difficult regions (as perceived by that specific user). Even more powerfully, it creates interpretable tree diagrams that clearly show which exercise parameters contribute most to the individual’s difficulty.
- Explainable Insights: Both the user and their caretakers can understand why a certain movement is challenging, fostering better communication and goal setting.
Key Benefits:
- Truly Personalized Rehab: Exercises can be adapted in real-time to provide the optimal challenge, maximizing both rehabilitation outcomes and user motivation.
- Increased Engagement: Game-like interactions become more effective when tailored to the individual’s unique abilities and challenges.
- Objective Progress Tracking: QTrobot provides precise data and visualizations to monitor rehabilitation progress over time.
- Actionable Insights: Clinicians gain a readily interpretable model of individual difficulty, enabling more targeted and effective therapy plans.
The Future: This research opens exciting possibilities for QTrobot to power adaptable and engaging rehabilitation exercises that truly cater to the individual needs of each user, paving the way for more effective and motivating recovery journeys.
Reference:
Modeling Personalized Difficulty of Rehabilitation Exercises Using Causal Trees