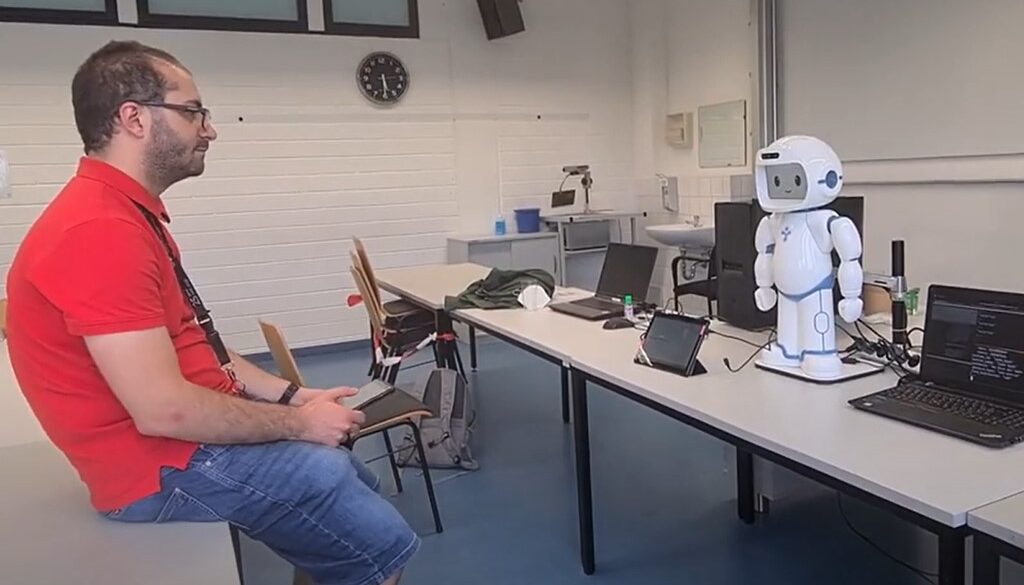
Abstract:
In traditional robot-assisted therapy sessions, robots often require direct control by therapists through methods like the Wizard-of-Oz protocol, which complicates sessions by preventing therapists from fully engaging with the patient. To address this, the project focuses on developing a learning-based behavioral model to enhance a robot’s autonomous decision-making capabilities. Researchers have explored the use of reinforcement learning for model training and evaluated various reward functions based on user engagement and activity performance. Additionally, they have examined strategies to streamline the learning process, including training the behavior model using a learned user model, transferring policies between different user groups, and incorporating expert feedback into policy learning.
Their findings indicate that transferring policies across user groups can significantly expedite the learning process, although the choice of reward function critically influences the robot’s potential actions. While our primary aim was to explore the personalization pipeline, we also conducted a preliminary real-world study with six participants engaging in a sequence learning game alongside an assistive robot. The initial results suggest that policies developed from guidance might optimize user engagement and game performance, but a larger user study is necessary to confirm these findings.
Reference:
Stolarz, Michał, et al. “Learning-based personalisation of robot behaviour for robot-assisted therapy.” Frontiers in Robotics and AI 11 (2024): 1352152.